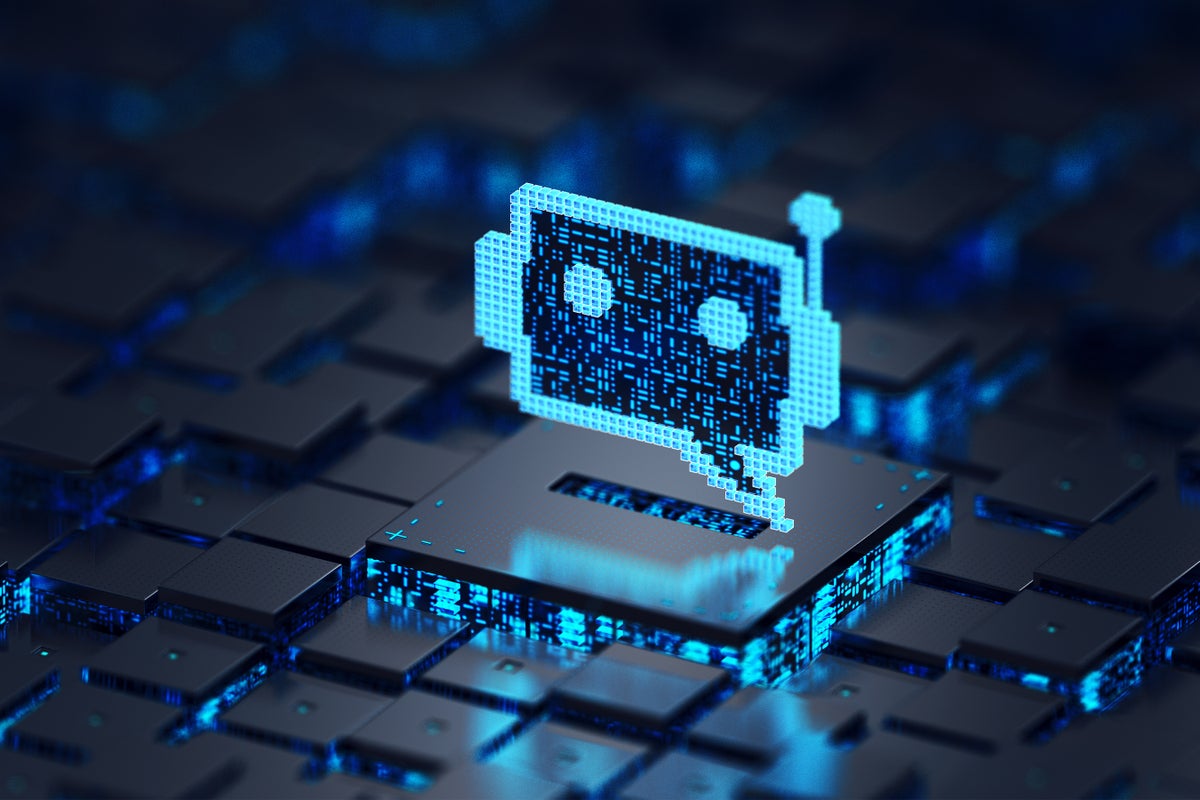
Few computer science breakthroughs have done so much in so little time as the artificial intelligence design known as a transformer. A transformer is a form of deep learning—a machine model based on networks in the brain—that researchers at Google first proposed in 2017. Seven years later the transformer, which enables ChatGPT and other chatbots to quickly generate sophisticated outputs in reply to user prompts, is the dynamo powering the ongoing AI boom. As remarkable as this AI design has already proved to be, what if you could run it on a quantum computer?
That might sound like some breathless mash-up proposed by an excitable tech investor. But quantum-computing researchers are now in fact asking this very question out of sheer curiosity and the relentless desire to make computers do new things. A new study published recently in Quantum used simple hardware to show that rudimentary quantum transformers could indeed work, hinting that more developed quantum-AI combinations might solve crucial problems in areas including encryption and chemistry—at least in theory.
A transformer’s superpower is its ability to discern which parts of its input are more important than others and how strongly those parts connect. Take the sentence “She is eating a green apple.” A transformer could pick out the sentence’s key words: “eating,” “green” and “apple.” Then, based on patterns identified in its training data, it would judge that the action “eating” has little to do with the color “green” but a great deal more to do with the object “apple.” Computer scientists call this feature an “attention mechanism,” meaning it pays the most attention to the most important words in a sentence, pixels in an image or proteins in a sequence. The attention mechanism mimics how humans process language, performing a task that is elementary for most young children but that—until the ChatGPT era—computers had struggled with.
On supporting science journalism
If you're enjoying this article, consider supporting our award-winning journalism by subscribing. By purchasing a subscription you are helping to ensure the future of impactful stories about the discoveries and ideas shaping our world today.
Attention mechanisms currently run on supercomputers with powerful processors, but they still use basic binary bits that hold values of either 0 or 1. Physicists describe these as “classical” machines, which also include smartphones and PCs. Quantum hardware, on the other hand, taps into the weirdness of quantum mechanics to solve problems too impractical for classical computers. That’s because quantum bits, aka qubits, can exist as a 0, a 1 or a spectrum of other possible states. So could developers build a superior attention mechanism using qubits? “Quantum computers are not expected to be a computational panacea, but we won’t know until we try,” says quantum computing researcher Christopher Ferrie at the University of Technology Sydney, who wasn’t involved with the new study.
An author of the study, Jonas Landman, had previously crafted quantum facsimiles of other brainlike AI designs to run on quantum hardware. “We wanted to look at transformers because they seemed to be the state of the art of deep learning,” says Landman, a quantum computing researcher at the University of Edinburgh and a computing firm called QC Ware. In the new research, he and his colleagues adapted a transformer designed for medical analysis. From a database of images of 1,600 people’s retinas, some in healthy eyes and some in people with diabetes-induced blindness, the quantum model sorted each image into one of five levels from no damage to the most severe.
Developing their quantum transformer was a three-step process. First, before even touching any quantum hardware, they needed to design a quantum circuit—a quantum program’s “code,” in other words—for a transformer. They made three versions, each of which could theoretically pay attention more efficiently than a classical transformer, as demonstrated by mathematical proofs.
Bolstered by confidence from the math, the study authors tested their designs on a quantum simulator—a qubit emulator that runs on classical hardware. Emulators avoid a problem plaguing today’s real quantum computers, which are still so sensitive to heat, electromagnetic waves and other interference that qubits can become muddled or entirely useless.
On the simulator, each quantum transformer categorized a set of retinal images with between 50 and 55 percent accuracy—greater than the 20 percent accuracy that randomly sorting retinas into one of five categories would have achieved. The 50- to 55-percent range was about the same accuracy level (53 to 56 percent) achieved by two classical transformers with vastly more complex networks.
Only after this could the scientists move on to the third step: operating their transformers on real IBM-made quantum computers, using up to six qubits at a time. The three quantum transformers still performed with between 45 and 55 percent accuracy.
Six qubits is not very many. For a viable quantum transformer to match the chatbot giants of Google’s Gemini or OpenAI’s ChatGPT, some researchers think computer scientists would have to create a code that uses hundreds of qubits. Quantum computers of that size already exist, but designing a comparatively colossal quantum transformer isn’t yet practical because of the interference and potential errors involved. (The researchers tried higher qubit numbers but did not see the same success.)
The group isn’t alone in its work on transformers. Last year researchers at IBM’s Thomas J. Watson Research Center proposed a quantum version of a transformer type known as a graph transformer. And in Australia, Ferrie’s group has designed its own transformer quantum circuit concept. That team is still working on the first step that QC Ware passed: mathematically testing the design before trying it out.
But suppose a reliable quantum computer existed—one with more than 1,000 qubits and where interference is somehow kept to a minimum. Would a quantum transformer, then, always have the advantage? Maybe not. Head-to-head comparisons between quantum and classical transformers are not the right approach because the two probably have different strengths.
For one thing, classical computers have the benefit of investment and familiarity. Even as quantum-computing technology matures, “It will take many years for quantum computers to scale up to that regime, and classical computers won’t stop growing in the meantime,” says Nathan Killoran, head of software at quantum computing firm Xanadu, who was not involved with the new research. “Classical machine learning is just so powerful and so well financed that it may just not be worth it to replace it entirely with an emerging technology like quantum computing in our lifetimes.”
Additionally, quantum computers and classical machine learning each excel at different kinds of problems. Modern deep-learning algorithms detect patterns within their training data. It’s possible that qubits can learn to encode the same patterns, but it is not clear if they are optimal for the task. That’s because qubits offer the greatest advantage when a problem is “unstructured,” meaning its data have no clear patterns to find in the first place. Imagine trying to find a name in a phone book with no alphabetization or order of any kind; a quantum computer can find that word in the square root of the time a classical computer would take.
But the two options are not exclusive. Many quantum researchers believe a quantum transformer’s ideal place will be as part of a classical-quantum hybrid system. Quantum computers could handle the trickier problems of chemistry and materials science, while a classical system crunches through volumes of data. Quantum systems might also prove valuable at generating data—decrypted cryptographic keys, for example, or the properties of materials that don’t yet exist, both of which are hard for classical computers to do—that could in turn help train classical transformers to perform tasks that now remain largely inaccessible.
And quantum transformers may bring other bonuses. Classical transformers, at the scales they are now being used, consume so much energy that U.S. utilities are keeping carbon-spewing coal plants operational just to meet new data centers’ power demands. The dream of a quantum transformer is also the dream of a leaner, more efficient machine that lightens the energy load.
"like this" - Google News
April 22, 2024 at 07:36PM
https://ift.tt/7hQeS1n
Quantum Computers Can Run Powerful AI That Works like the Brain - Scientific American
"like this" - Google News
https://ift.tt/CXVT8aE
Shoes Man Tutorial
Pos News Update
Meme Update
Korean Entertainment News
Japan News Update
No comments:
Post a Comment